Have you ever wondered how self-driving cars make decisions on the road? Or how AI can handle customer service without human intervention? Thanks to AI agents powering autonomous systems.
For business owners, this technology is a game-changer. From automating repetitive tasks to making real-time decisions, AI agents can simplify operations and boost productivity. But here’s the catch: to take full advantage of this tech, you need the right platform.
In this blog, we’ll walk you through the top AI agent platforms that can help you build the autonomous systems your business needs. Want to know which platform can help you save time, cut costs, and scale effectively? Keep reading to find out!
What are Autonomous Systems?
Autonomous systems are technologies designed to perform tasks or make decisions independently, without the need for human intervention. These systems use data, algorithms, and machine learning to analyze information, make informed decisions, and take action on their own. They operate based on predefined rules, real-time data, and even adapt to changing circumstances over time.
In simple terms, autonomous systems can think and act on their own, which makes them ideal for tasks that require consistency, speed, and accuracy. For business owners, adopting such systems can significantly enhance operational efficiency and reduce reliance on manual labor.
The Role of AI Agents in Autonomy
AI agents are at the heart of autonomous systems. They allow machines to perform tasks and make decisions on their own, without human help. These agents use data, algorithms, and learning to improve their performance over time.
Here’s how AI agents enable autonomy:
- Making Decisions: AI agents quickly analyze data and make decisions faster than humans. This is crucial for autonomous systems like self-driving cars or automated financial trading, where decisions must be made in real-time without delays.
- Learning Over Time: AI agents learn from their previous actions and improve over time. For autonomous systems, this means the more they interact with their environment, the better they get at making decisions, whether it’s optimizing a supply chain or personalizing a customer’s shopping experience.
- Automating Tasks: AI agents can take over routine tasks like responding to customer queries or managing inventory. This is what makes systems autonomous, as they can operate independently without human intervention, saving businesses valuable time and effort.
- Improving Efficiency: AI agents handle tasks accurately and faster, reducing the chance of errors. For businesses, this means fewer mistakes, quicker processes, and a smoother workflow in everything from customer support to back-end operations like data entry or order management.
Also Read: Understanding AI Agents: A Comprehensive Guide
By using AI agents, businesses can increase efficiency, cut costs, and let their systems work independently.
Types of AI Agents
AI agents come in various forms, each with its unique capabilities, designed to perform specific tasks within autonomous systems. Below are some of the most popular types of AI agents used in building such systems:
Reactive Agents
These agents respond to the current environment without any memory of past interactions. They are typically designed for simple, real-time tasks that require immediate action based on sensory input. Examples include robots used for repetitive tasks or basic AI in video games.
Deliberative Agents
Deliberative agents are more complex and take time to plan their actions. They have an internal model of the world and use reasoning to make decisions. These agents are ideal for scenarios where long-term planning is necessary, such as autonomous vehicles that must navigate complex environments.
Learning Agents
Learning agents improve their performance over time by gaining experience. Through reinforcement learning or supervised learning, these agents adapt to new situations and optimize their actions. They are frequently used in systems like recommendation engines or smart assistants.
Collaborative Agents
These agents are designed to work together, sharing information and resources to achieve a common goal. In autonomous systems, they often collaborate with other agents or human operators. Examples include drones working together for surveillance or robots in industrial environments.
Autonomous Agents
Autonomous agents are capable of making decisions and taking actions without human intervention. They utilize a combination of sensory data, learning, and planning to adapt and function independently in dynamic environments. Self-driving cars and autonomous delivery robots are prime examples of this agent type.
Now, let’s take a look at leading platforms that can help you build these powerful systems.
Leading AI Agent Platforms in 2025 for Building Autonomous Systems
In 2025, AI agent platforms are the backbone of many autonomous systems, driving businesses toward greater efficiency and innovation. These platforms enable businesses to build autonomous systems that can think, act, and adapt independently.
Whether it’s self-driving cars, automated customer support, or supply chain management, AI agents are the key players in shaping the future of autonomy.
Here’s a look at the top AI agent platforms for building autonomous systems in 2025:
1. CrewAI
CrewAI is designed to build intelligent, multi-agent systems that collaborate and adapt in real-time, making it ideal for creating autonomous systems in industries like logistics and customer service.
Key Features:
- Collaborative Multi-Agent Systems: CrewAI enables multiple AI agents to work together autonomously, optimizing tasks and decision-making.
- Real-Time Adaptation: Agents adapt to changing environments in real-time, which is crucial for autonomous systems that require continuous learning and adjustment.
- Seamless Integration: Easily integrates with existing business systems like CRMs and enterprise software to automate operations.
- Scalable Architecture: CrewAI’s platform supports scaling from small projects to enterprise-level autonomous systems.
- Data-Driven Decisions: AI agents make decisions based on vast datasets, enabling high-level automation without human intervention.
Use Cases and Applications:
- Autonomous Customer Support: AI agents handle customer queries, learn from past interactions, and continuously improve responses.
- Supply Chain Automation: Automating inventory management, order processing, and logistics without manual oversight.
Also Read: Top AI Agent Frameworks for Effective Automation
2. AutoGen
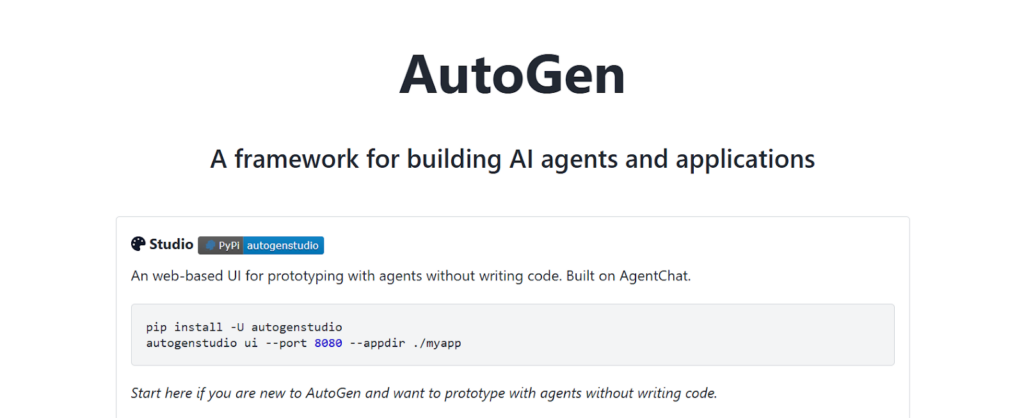
AutoGen stands out for its predictive analytics and machine learning capabilities, enabling businesses to create autonomous systems that can make complex decisions in finance, healthcare, and retail.
Key Features:
- Predictive Decision-Making: AutoGen’s AI agents predict future outcomes based on historical data, essential for systems that need foresight, like autonomous trading or inventory management.
- Automated Workflows: It automates entire workflows, reducing the need for human involvement and ensuring continuous operation.
- Custom Algorithms: The platform allows businesses to build their own machine learning models for specific needs, offering flexibility in system design.
- Integration with Legacy Systems: AutoGen can easily integrate with existing infrastructure, making it ideal for businesses looking to automate without overhauling current systems.
- Continuous Learning: AI agents improve over time, adapting to new data and refining their decision-making processes for more accurate results.
Use Cases and Applications:
- Autonomous Financial Trading: AI agents analyze market trends and execute trades independently, maximizing profit potential.
- Healthcare Diagnostics: AI agents autonomously analyze patient data and provide diagnostic recommendations, reducing the need for manual review.
3. LangChain
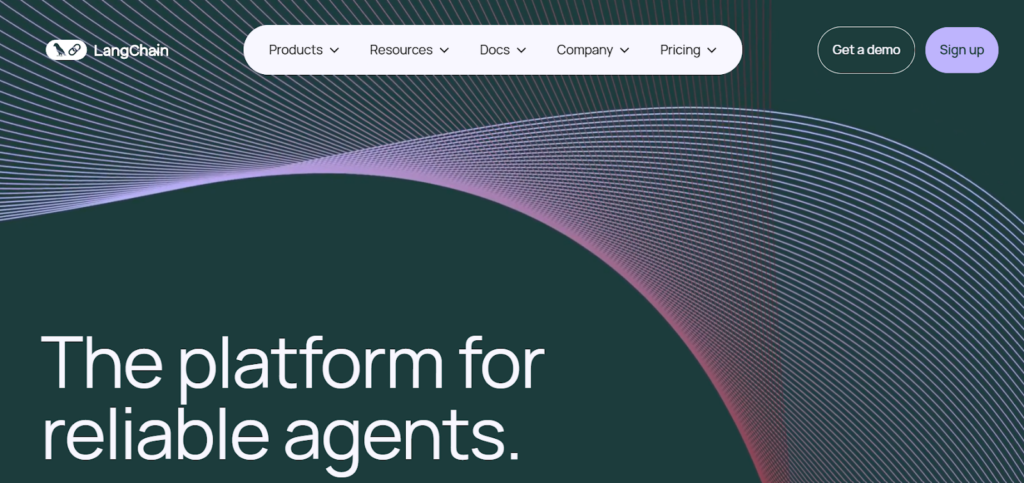
LangChain is a platform that specializes in building natural language processing (NLP) agents, which are essential for creating autonomous systems that interact intelligently with humans.
Key Features:
- Advanced NLP Capabilities: LangChain’s agents understand and respond to human language, making it perfect for conversational AI systems in customer service.
- Multi-Task Automation: These agents can handle a wide range of tasks simultaneously, making them ideal for managing diverse business operations autonomously.
- Contextual Awareness: LangChain’s agents remember context and past interactions, allowing for smooth, long-term autonomous communication with customers.
- Cross-Platform Functionality: Integrates seamlessly across different communication platforms like email, chat, and social media.
- Real-Time Learning: The platform allows AI agents to learn from ongoing conversations, improving their responses as they go.
Use Cases and Applications:
- Autonomous Chatbots: LangChain powers intelligent chatbots that can autonomously handle customer inquiries, provide recommendations, and solve problems.
- Automated Virtual Assistants: AI agents act as virtual assistants, managing tasks, scheduling, and responding to emails without human intervention.
If you’re new to building AI agents, start with our beginner-friendly guide that walks through architecture basics, use cases, and frameworks.
4. Vertex AI Agent Builder
Developed by Google, Vertex AI Agent Builder is a powerful tool for businesses looking to deploy autonomous systems quickly. It’s built for flexibility, allowing organizations to build complex AI models for various industries.
Key Features:
- Pre-Built Models: Provides access to pre-trained models that businesses can use to jumpstart their autonomous systems, saving time in development.
- Customizable AI Agents: Offers customizable templates to build AI agents tailored to specific business needs, supporting a wide range of autonomous tasks.
- Cloud Integration: Fully integrates with Google Cloud, ensuring seamless scalability for enterprise-level autonomous systems.
- End-to-End Support: From development to deployment, Vertex AI provides tools to manage every step of the process for building autonomous systems.
- Advanced Machine Learning Tools: Includes cutting-edge tools for machine learning, such as reinforcement learning and deep learning, essential for creating sophisticated autonomous systems.
Use Cases and Applications:
- Smart Manufacturing: AI agents autonomously manage production lines, monitor quality, and optimize manufacturing processes in real-time.
- Autonomous Supply Chain Management: From order processing to delivery, AI agents manage the entire supply chain autonomously, reducing human oversight.
5. Cogniflow
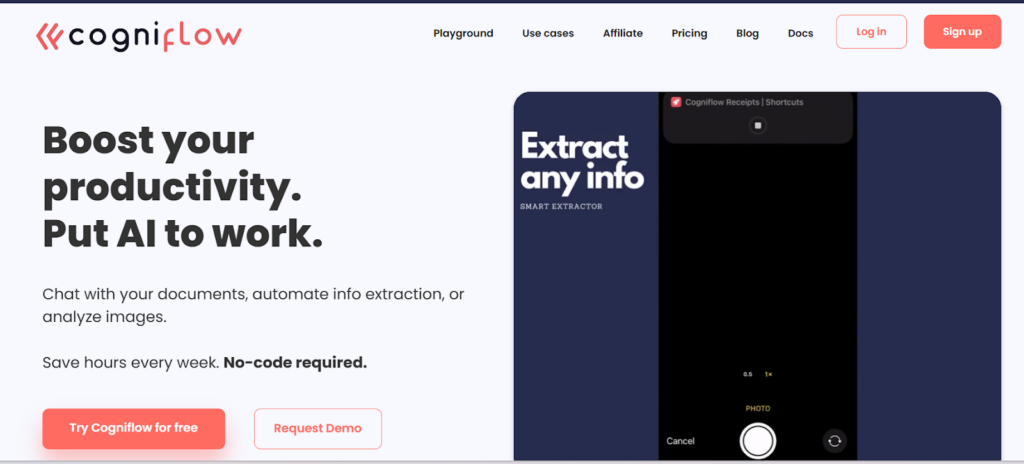
Cogniflow specializes in building adaptive autonomous systems that learn from data and continuously improve their performance, making it ideal for industries like healthcare, finance, and retail.
Key Features:
- Self-Learning AI Agents: Cogniflow agents adapt to new data over time, improving their performance and decision-making abilities autonomously.
- Comprehensive AI Models: The platform offers a range of AI models that can handle everything from decision-making to data analysis and forecasting.
- Simple User Interface: Despite its complex capabilities, Cogniflow provides an intuitive interface, allowing businesses to build autonomous systems without deep technical expertise.
- End-to-End Automation: From data processing to decision-making, Cogniflow’s agents automate entire workflows, eliminating manual tasks.
- Security and Compliance: The platform is designed with a strong focus on security, ensuring that autonomous systems operate safely and in compliance with industry regulations.
Use Cases and Applications:
- Autonomous Risk Management: AI agents autonomously assess financial risks and provide recommendations for mitigation.
- Healthcare Automation: Agents analyze patient data, predict medical conditions, and assist in decision-making for doctors and healthcare providers.
6. H2O.ai
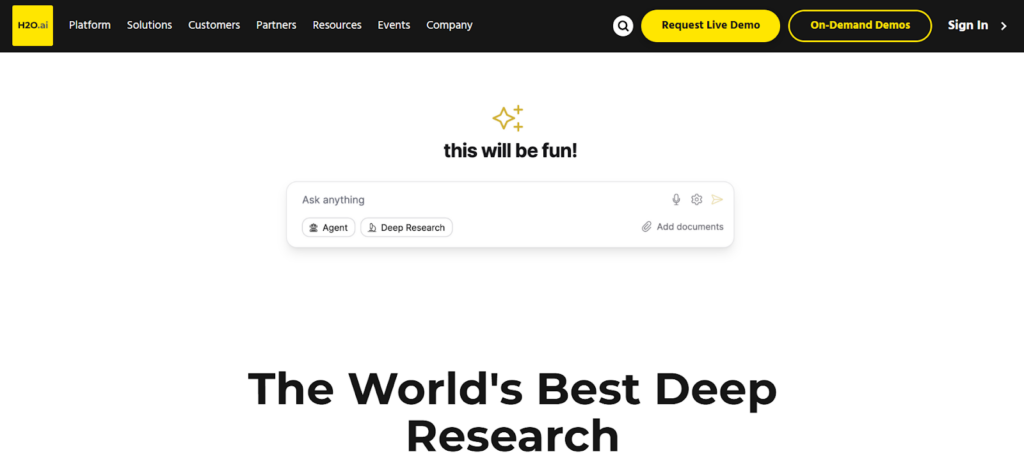
H2O.ai is a leading platform for creating autonomous systems using machine learning and artificial intelligence. It’s known for making AI accessible to businesses without requiring deep technical expertise, which is crucial for non-tech-driven industries looking to implement autonomous systems.
Key Features:
- AutoML Capabilities: H2O.ai simplifies the creation of machine learning models with automated tools, making it easy for businesses to build autonomous systems with minimal effort.
- Scalable AI Models: The platform supports large-scale machine learning models, allowing businesses to handle vast amounts of data and deploy autonomous systems at scale.
- Data Processing Power: With its powerful data processing capabilities, H2O.ai can quickly analyze large datasets, providing the foundation for autonomous decision-making systems.
- Advanced Visualization: It offers visual tools for understanding complex models, helping businesses grasp how their autonomous systems work.
- Open-Source: H2O.ai is an open-source platform, making it flexible and customizable to suit specific business needs.
Use Cases and Applications:
- Customer Segmentation: Automating the classification of customers into segments for personalized marketing campaigns.
- Predictive Maintenance: Using AI agents to monitor equipment, predict failures, and automatically trigger maintenance requests.
- Real-Time Fraud Detection: AI agents detect unusual activity in financial transactions and autonomously flag potential fraud.
7. IBM Watson
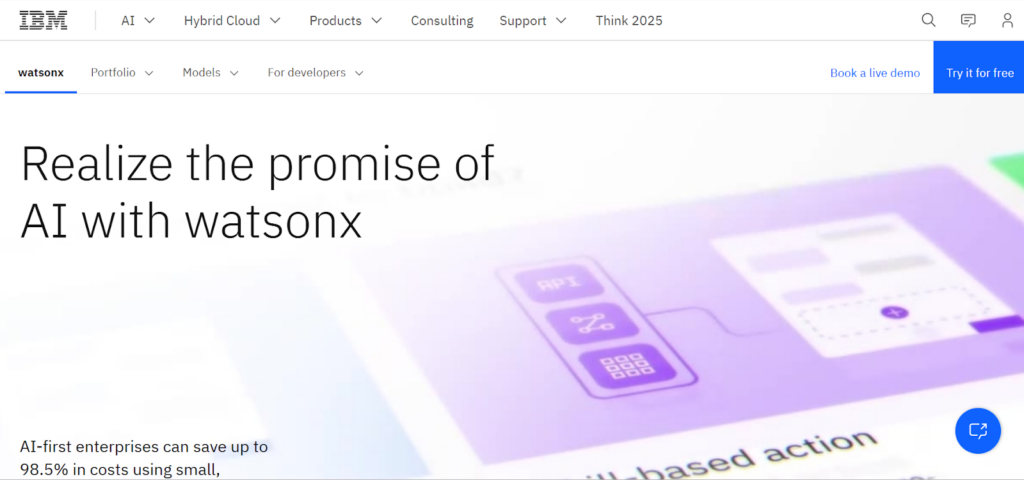
IBM Watson is a leading AI platform that powers autonomous systems with its cognitive capabilities, enabling businesses to build AI agents capable of understanding natural language, analyzing data, and making decisions without human intervention.
Key Features:
- Natural Language Processing (NLP): Watson’s NLP capabilities make it ideal for creating conversational AI agents that can understand and respond to human language.
- Machine Learning Models: Businesses can leverage Watson’s pre-built machine learning models or create custom models to suit specific needs in their autonomous systems.
- Integration with Enterprise Systems: Watson integrates seamlessly with existing business systems, such as CRM and ERP tools, enabling full autonomy across business processes.
- Predictive Insights: Watson’s AI agents analyze historical data to predict future outcomes, helping businesses make proactive decisions.
- Automated Decision-Making: Watson’s AI agents can make autonomous decisions in real-time, reducing the need for human oversight.
Use Cases and Applications:
- Autonomous Customer Support: Watson powers chatbots and virtual assistants that handle customer interactions autonomously, providing personalized responses.
- Supply Chain Automation: AI agents use predictive insights to optimize inventory, manage shipments, and automate ordering processes in real-time.
- Healthcare AI: Watson helps medical professionals make faster decisions by analyzing patient data and suggesting treatment options autonomously.
8. OpenAI GPT-4 (Agent-Based Systems)
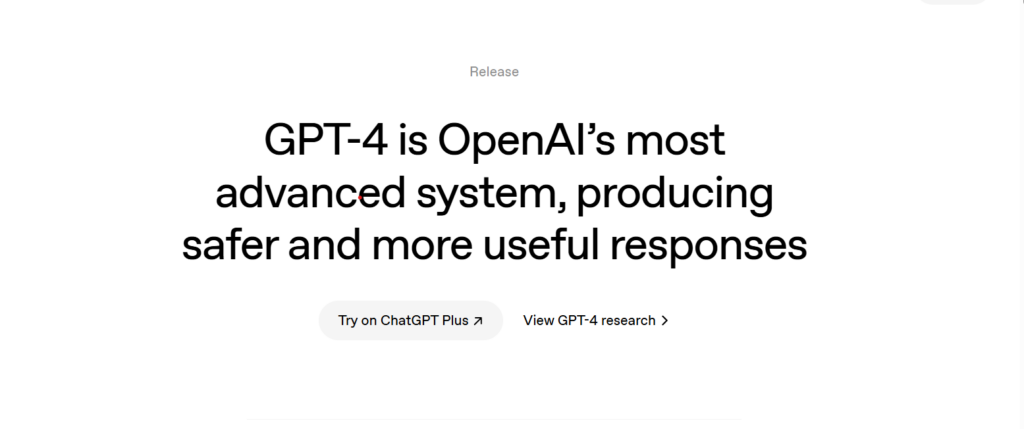
OpenAI’s GPT-4 is a state-of-the-art platform that enables businesses to build sophisticated autonomous systems powered by conversational AI. While GPT-4 is widely recognized for its ability to generate human-like text, its real strength lies in its ability to power AI agents that can handle complex, context-rich conversations and tasks.
Key Features:
- Contextual Understanding: GPT-4 can understand and respond to context, making it perfect for building autonomous systems that need to engage in nuanced decision-making.
- Multitasking: This platform can manage multiple tasks simultaneously, allowing for the creation of multi-agent systems that work together autonomously.
- Customizable Outputs: Businesses can tailor GPT-4’s responses to fit specific business needs, such as creating personalized customer experiences or automated internal workflows.
- Extensive Knowledge Base: GPT-4’s access to vast amounts of data enables it to generate accurate and intelligent decisions in a wide range of fields.
- Integration with Other AI Tools: GPT-4 seamlessly integrates with other AI tools, providing a cohesive environment for building advanced autonomous systems.
Use Cases and Applications:
- Intelligent Virtual Assistants: Automating administrative tasks, scheduling, and customer support using AI-powered virtual assistants.
- Content Generation: GPT-4 can autonomously create content for marketing, product descriptions, or even code, depending on the business needs.
- Autonomous Decision Support: GPT-4 powers AI agents that assist businesses in making complex decisions, such as strategic planning or resource allocation.
9. DeepMind (Google AI)
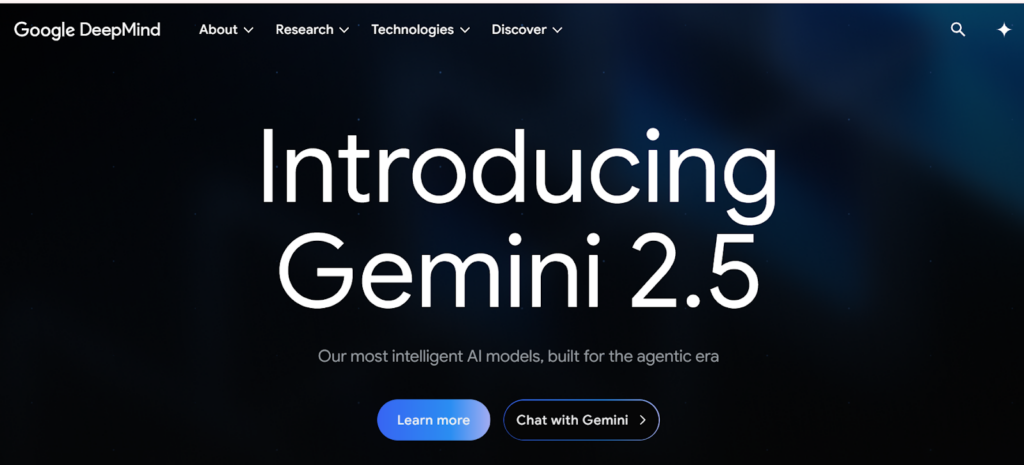
DeepMind, a subsidiary of Google, is renowned for its cutting-edge work in artificial intelligence. The platform is used to create autonomous systems capable of learning and performing tasks with little to no human supervision. DeepMind focuses on advancing AI to tackle complex problems in areas like healthcare, energy efficiency, and robotics.
Key Features:
- Reinforcement Learning: DeepMind utilizes reinforcement learning to build AI agents that can learn from experience and make better decisions over time.
- Simulated Environments: It creates simulated environments to train AI agents, improving their decision-making in the real world.
- Adaptive Systems: DeepMind’s AI agents can adapt to new challenges and scenarios, making them ideal for dynamic environments where change is constant.
- Predictive Algorithms: The platform uses predictive algorithms to anticipate future outcomes, improving the ability of autonomous systems to act preemptively.
- High-Performance Computing: DeepMind leverages Google’s advanced computing resources, enabling it to run large-scale autonomous systems that require immense computational power.
Use Cases and Applications:
- Healthcare Automation: DeepMind is used to build systems that can autonomously analyze medical images, predict diseases, and suggest treatments.
- Robotics: AI agents powered by DeepMind autonomously control robots, from basic tasks like assembly line work to more complex activities like navigation in unknown environments.
- Energy Efficiency: DeepMind’s AI agents optimize energy consumption in data centers, reducing costs and improving sustainability.
10. Microsoft Azure AI
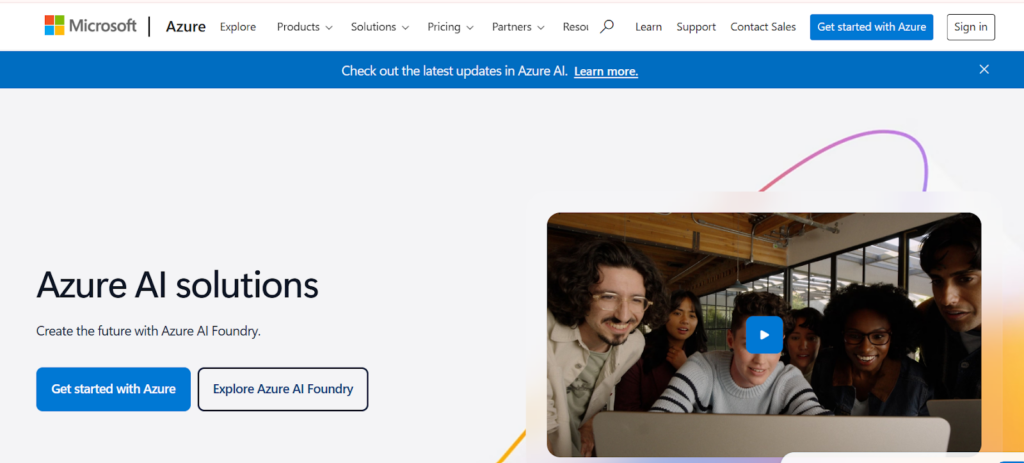
Microsoft’s Azure AI platform offers businesses a comprehensive suite of tools for building autonomous systems, with an emphasis on scalability and ease of integration. Azure AI helps businesses automate processes using AI agents that are easy to deploy and manage.
Key Features:
- End-to-End AI Tools: Azure AI provides tools for every part of the AI lifecycle, from training models to deploying them in real-time autonomous systems.
- Integrated with Azure Cloud: The platform integrates with Microsoft Azure’s cloud services, allowing businesses to scale their AI-powered systems effortlessly.
- Pre-trained Models: Azure AI offers pre-built models for tasks like computer vision, natural language processing, and anomaly detection.
- Customizable AI: Users can customize models to suit their specific needs, building more tailored autonomous systems.
- Security and Compliance: Azure AI prioritizes security, ensuring that AI systems are deployed safely and in compliance with regulations.
Use Cases and Applications:
- Retail Automation: Automating inventory management, sales forecasting, and customer interactions using AI agents.
- Predictive Analytics: Using AI agents to forecast trends in data, improving decision-making in fields like finance and marketing.
- IoT Device Management: Managing and optimizing networks of IoT devices autonomously, such as smart homes or industrial equipment.
These AI agent platforms give businesses the tools to build autonomous systems that work on their own, learn over time, and adjust to new situations.
Autonomous systems save time, cut costs, and work 24/7—but how?
See real-world examples here and build yours now!
Building autonomous systems brings many benefits, but it also comes with challenges. From technical hurdles to ethical concerns, businesses encounter a variety of obstacles in creating systems that function smoothly and securely. However, with the right steps, these challenges can be effectively addressed.
Steps to Build an Autonomous System Using AI Agent Platforms
Building an autonomous system using AI agent platforms involves several key steps. Here’s a clear, step-by-step guide to help you understand the process:
1. Define the Problem and Objectives
Start by identifying the problem your autonomous system will solve. Clearly define the objectives and scope of the system, such as whether it’s for navigation, decision-making, or process automation. This will help guide the design and selection of AI agents.
2. Select the Right AI Agent Platform
Choose an AI agent platform that aligns with your system’s requirements. Popular platforms like OpenAI, Google AI, or Microsoft Azure provide various tools for building agents. Consider the platform’s scalability, flexibility, and support for the type of AI agents you need (reactive, deliberative, or learning agents).
3. Design the AI Agent Architecture
Plan the structure of your AI agents. This includes determining how they will interact with the environment, the data they will process, and how they will make decisions. Ensure the architecture supports seamless integration with other components of the autonomous system.
4. Integrate Sensors and Data Sources
Autonomous systems rely heavily on data from sensors, cameras, and other inputs. Integrate relevant data sources into your system to allow the AI agents to perceive and respond to their environment. This may include LiDAR, GPS, or real-time video feeds.
5. Train the AI Agents
Use machine learning or reinforcement learning techniques to train your AI agents. Provide them with the necessary data, and allow them to learn from past experiences. This step is critical for improving the performance and adaptability of the system.
6. Test and Validate the System
Testing is crucial to ensure that your autonomous system performs as expected. Simulate real-world scenarios and monitor the AI agents’ responses to different situations. Make adjustments based on performance to ensure safety and reliability.
7. Deploy and Monitor
Once the system is tested, deploy it in the intended environment. Continuous monitoring is essential to ensure the AI agents adapt to new conditions and remain effective. Use feedback loops to retrain the agents if necessary and improve performance over time.
8. Maintain and Optimize
Autonomous systems require ongoing maintenance and optimization. Regularly update the platform, monitor for potential issues, and make performance improvements based on data and user feedback.
While these steps lay the foundation for building autonomous systems, it’s important to recognize the challenges that can arise along the way.
Challenges and Solutions in Building Autonomous Systems
Let’s explore some of the main challenges businesses face when building autonomous systems and the solutions that can help overcome them.
1. Data Quality and Availability
One of the biggest challenges in building autonomous systems is ensuring access to clean, high-quality data. AI agents rely on vast amounts of data to learn and make decisions, and if that data is incomplete, outdated, or biased, the system will not perform as expected. For instance, in autonomous vehicles, data from sensors and cameras must be precise to avoid accidents.
Solution: Businesses can invest in robust data collection systems, ensuring they gather accurate and relevant data. Data cleaning and preprocessing tools can also help improve data quality. Additionally, utilizing data augmentation techniques or working with third-party data providers can fill gaps in data availability.
2. Integration with Existing Systems
Many businesses have legacy systems in place, and integrating new autonomous systems with these older technologies can be complex. Whether it’s an AI-powered supply chain system or an automated customer support agent, ensuring smooth integration with existing software, tools, and processes is essential but challenging.
Solution: Businesses can use modular architectures and APIs to make integration easier. Adopting platforms that support plug-and-play features and allow for smooth communication between new and old systems can significantly reduce the complexity of this process.
3. Real-Time Decision Making
For many autonomous systems, such as self-driving cars or real-time financial trading algorithms, the ability to make decisions in real-time is critical. Even a slight delay in decision-making can result in failure or missed opportunities, making real-time processing a challenging aspect of building autonomous systems.
Solution: Edge computing can help process data locally, reducing latency. Investing in faster processors and optimizing algorithms to handle data more efficiently can also make real-time decision-making more feasible.
4. Ensuring Security and Privacy
Autonomous systems often work with sensitive data, whether it’s personal information in customer interactions or critical operational data. Ensuring the security and privacy of this data is a significant concern, especially with the growing risk of cyberattacks.
Solution: Businesses must prioritize cybersecurity by adopting encryption methods, secure communication channels, and regular security audits. Additionally, AI models should be tested for vulnerabilities, and businesses should ensure compliance with data protection regulations (such as GDPR) to protect privacy.
5. Bias and Ethical Concerns
AI agents learn from historical data, which can sometimes carry biases. If these biases aren’t addressed, autonomous systems could reinforce inequalities, leading to unfair decisions. This is particularly problematic in industries like hiring, loan approvals, and healthcare, where AI decisions can significantly impact individuals’ lives.
Solution: To address biases, businesses should audit their data regularly, looking for and removing any skewed patterns. Transparency in decision-making processes is also essential, allowing stakeholders to understand how decisions are made. Implementing fairness algorithms that adjust for bias can help create more ethical systems.
6. Lack of Human Oversight
As autonomous systems become more advanced, the role of human oversight may decrease. However, entirely removing humans from the loop can be risky, especially in cases where decisions made by AI agents could have serious consequences, such as in healthcare or criminal justice.
Solution: A hybrid model, where humans still oversee AI-driven decisions, is a balanced approach. This model can include periodic checks and validation steps to ensure AI agents are performing as expected. Providing transparency in how autonomous systems make decisions can also help in building trust and maintaining oversight.
7. Scalability and Maintenance
Scaling autonomous systems to meet growing demands or adjusting them to new environments can be tricky. A system that works well in one context may face difficulties when applied to a broader scale or different conditions. Additionally, regular maintenance is crucial to ensure these systems continue functioning optimally.
Solution: Businesses can adopt modular, scalable systems that are easy to adjust as needs change. Regular updates and AI model retraining are necessary to ensure systems stay relevant and efficient. Automation of maintenance tasks can also reduce the effort needed to keep systems running smoothly.
How Codewave can Help You Build Autonomous Systems
There will definitely be challenges in building autonomous systems. But with experts like Codewave by your side, these challenges can be tackled efficiently. Here’s how:
- Data Quality Issues: While AI systems rely on clean and accurate data, Codewave ensures your data is processed and optimized for the best results.
- Integration with Existing Systems: Codewave helps integrate new autonomous systems with your existing infrastructure, ensuring a smooth transition without disruptions.
- Real-Time Decision Making: With Codewave’s expertise in real-time processing, your systems can make quick, accurate decisions as needed.
- Security and Privacy Concerns: Codewave prioritizes data security, making sure your systems are safe and compliant with privacy regulations.
- Scalability and Maintenance: Codewave ensures that your autonomous systems can scale with your business and remain effective over time.
With Codewave’s expertise and the right technology tools, your business can overcome these challenges and build a successful autonomous system.
Build Your Autonomous System Today
Up Next: Top AI Agents Transforming Business Productivity in 2025